Channel pruning¶
Context¶
Channel pruning (CP) is a model compression technique that removes less-important input channels from layers. AIMET supports channel pruning of 2D convolution (Conv2D) layers.
Procedure¶
The following figure illustrates the different steps in channel pruning a layer. These steps are repeated for all layers selected for compression in order of occurrence from the top of the model.
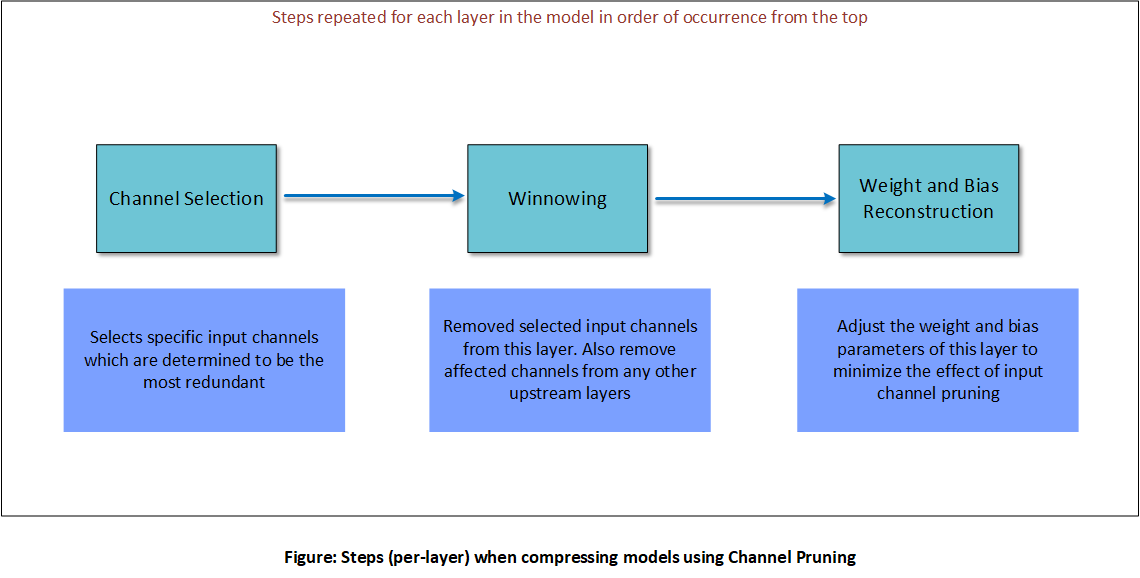
The steps are explained below.
Channel selection¶
For a layer and a specified compression ratio, channel selection analyzes the magnitude of each input channel based its kernel weights. It chooses the lowest-magnitude channels to be pruned.
Winnowing¶
Winnowing is the process of removing the input channels identified in channel selection, resulting in compressed tensors:
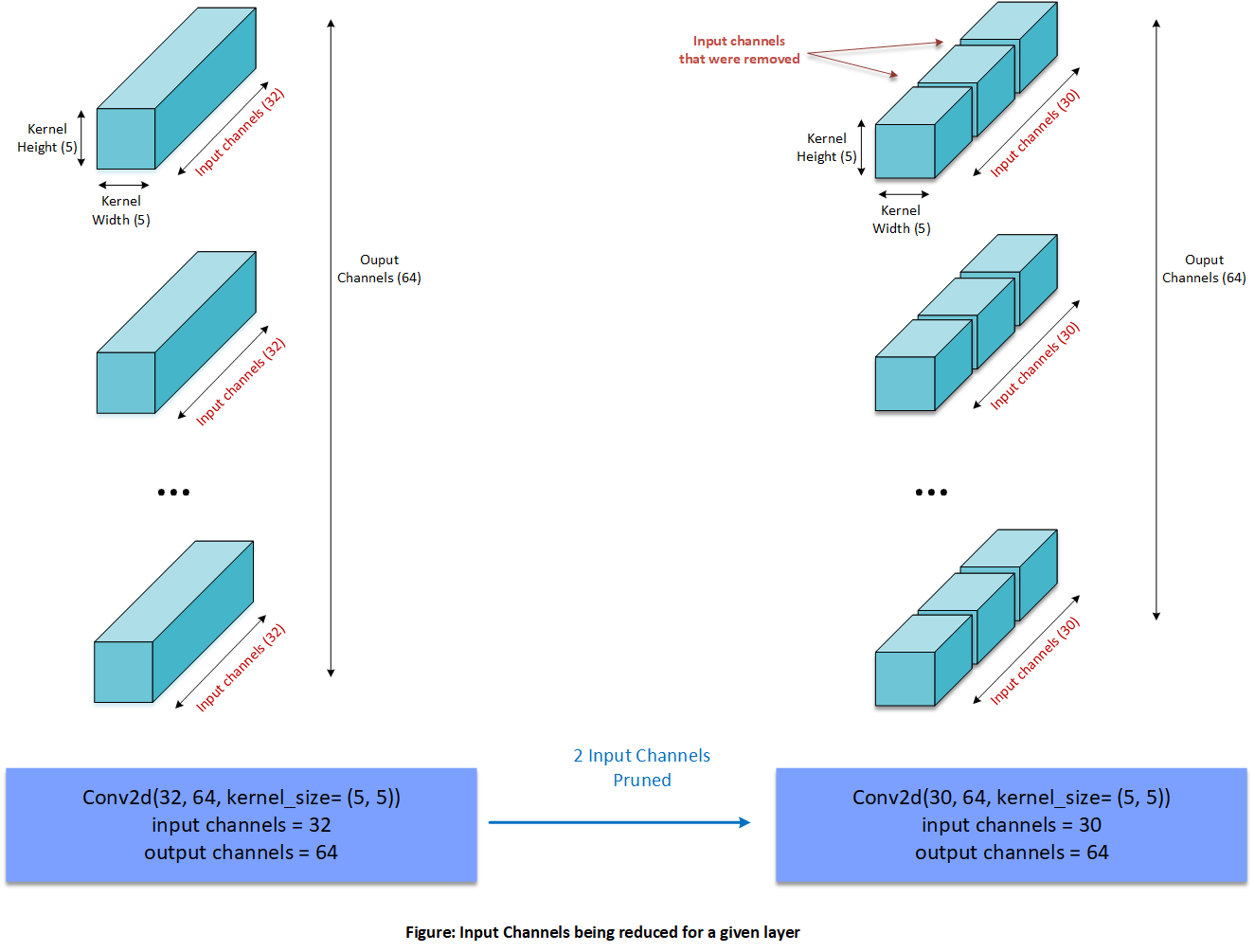
Once one or more input channels of a layer are removed, corresponding output channels of an upstream layer are also be removed to gain further compression. Skip-connections or residuals sometimes prevent upstream layers from being output-pruned.
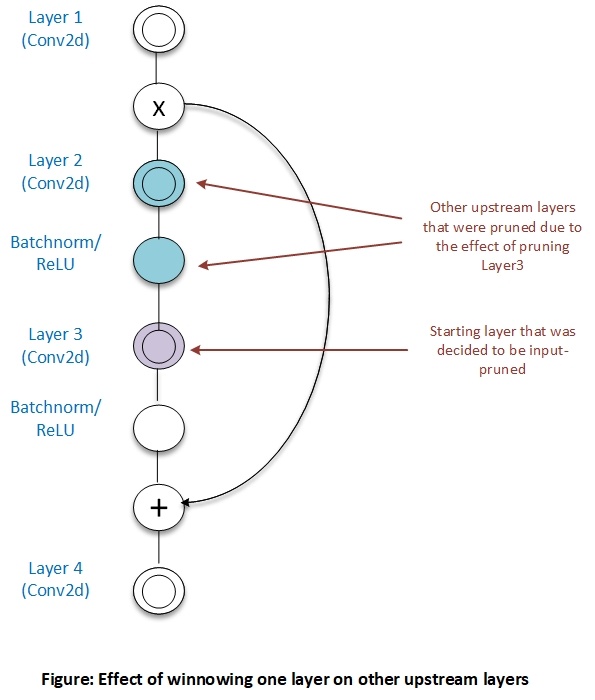
For more details on winnowing, see Winnowing.
Weight reconstruction¶
The final step in CP is to adjust the weight and bias parameters of a pruned layer to try and match pre-pruning output values. AIMET does this by performing linear regression on random samples of the layer’s input from the pruned model against corresponding output from the original model.
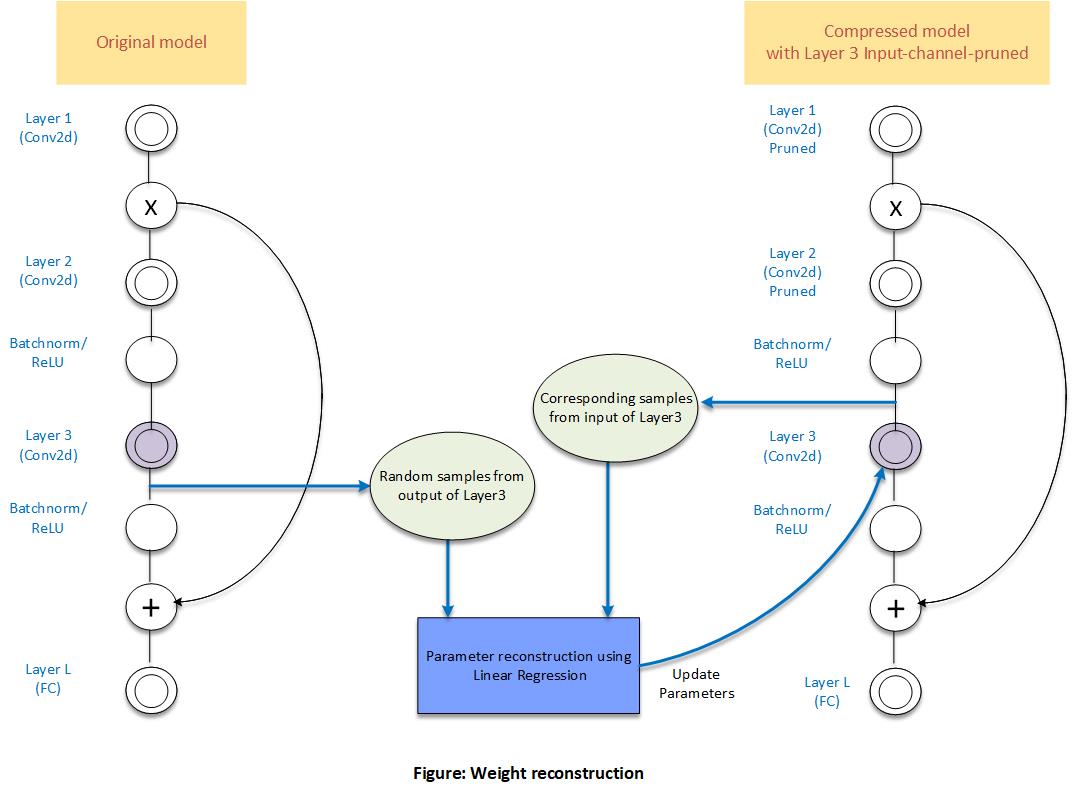
Workflow¶
Code example¶
Setup¶
import os
from decimal import Decimal
import torch
# Compression-related imports
from aimet_common.defs import CostMetric, CompressionScheme, GreedySelectionParameters, RankSelectScheme
from aimet_torch.defs import WeightSvdParameters, SpatialSvdParameters, ChannelPruningParameters, \
ModuleCompRatioPair
from aimet_torch.compress import ModelCompressor
def evaluate_model(model: torch.nn.Module, eval_iterations: int, use_cuda: bool = False) -> float:
"""
This is intended to be the user-defined model evaluation function.
AIMET requires the above signature. So if the user's eval function does not
match this signature, please create a simple wrapper.
Note: Honoring the number of iterations is not absolutely necessary.
However if all evaluations run over an entire epoch of validation data,
the runtime for AIMET compression will obviously be higher.
:param model: Model to evaluate
:param eval_iterations: Number of iterations to use for evaluation.
None for entire epoch.
:param use_cuda: If true, evaluate using gpu acceleration
:return: single float number (accuracy) representing model's performance
"""
return .5
Compression using Channel Pruning¶
Compressing using Channel Pruning in auto mode.
def channel_pruning_auto_mode():
# Load trained MNIST model
model = torch.load(os.path.join('../', 'data', 'mnist_trained_on_GPU.pth'))
# Specify the necessary parameters
greedy_params = GreedySelectionParameters(target_comp_ratio=Decimal(0.8),
num_comp_ratio_candidates=10)
auto_params = ChannelPruningParameters.AutoModeParams(greedy_params,
modules_to_ignore=[model.conv1])
data_loader = mnist_torch_model.DataLoaderMnist(cuda=True, seed=1, shuffle=True)
params = ChannelPruningParameters(data_loader=data_loader.train_loader,
num_reconstruction_samples=500,
allow_custom_downsample_ops=True,
mode=ChannelPruningParameters.Mode.auto,
params=auto_params)
# Single call to compress the model
results = ModelCompressor.compress_model(model,
eval_callback=evaluate_model,
eval_iterations=1000,
input_shape=(1, 1, 28, 28),
compress_scheme=CompressionScheme.channel_pruning,
cost_metric=CostMetric.mac,
parameters=params)
compressed_model, stats = results
print(compressed_model)
print(stats) # Stats object can be pretty-printed easily
Compressing using Channel Pruning in manual mode.
def channel_pruning_manual_mode():
# Load a trained MNIST model
model = torch.load(os.path.join('../', 'data', 'mnist_trained_on_GPU.pth'))
# Specify the necessary parameters
manual_params = ChannelPruningParameters.ManualModeParams([ModuleCompRatioPair(model.conv2, 0.4)])
data_loader = mnist_torch_model.DataLoaderMnist(cuda=True, seed=1, shuffle=True)
params = ChannelPruningParameters(data_loader=data_loader.train_loader,
num_reconstruction_samples=500,
allow_custom_downsample_ops=True,
mode=ChannelPruningParameters.Mode.manual,
params=manual_params)
# Single call to compress the model
results = ModelCompressor.compress_model(model,
eval_callback=evaluate_model,
eval_iterations=1000,
input_shape=(1, 1, 28, 28),
compress_scheme=CompressionScheme.channel_pruning,
cost_metric=CostMetric.mac,
parameters=params)
compressed_model, stats = results
print(compressed_model)
print(stats) # Stats object can be pretty-printed easily
API¶
Top-level API for Compression
- class aimet_torch.compress.ModelCompressor[source]
AIMET model compressor: Enables model compression using various schemes
- static ModelCompressor.compress_model(model, eval_callback, eval_iterations, input_shape, compress_scheme, cost_metric, parameters, trainer=None, visualization_url=None)[source]
Compress a given model using the specified parameters
- Parameters:
model (
Module
) – Model to compresseval_callback (
Callable
[[Any
,Optional
[int
],bool
],float
]) – Evaluation callback. Expected signature is evaluate(model, iterations, use_cuda). Expected to return an accuracy metric.eval_iterations – Iterations to run evaluation for
trainer – Training Class: Contains a callable, train_model, which takes model, layer which is being fine tuned and an optional parameter train_flag as a parameter None: If per layer fine tuning is not required while creating the final compressed model
input_shape (
Tuple
) – Shape of the input tensor for modelcompress_scheme (
CompressionScheme
) – Compression scheme. See the enum for allowed valuescost_metric (
CostMetric
) – Cost metric to use for the compression-ratio (either mac or memory)parameters (
Union
[SpatialSvdParameters
,WeightSvdParameters
,ChannelPruningParameters
]) – Compression parameters specific to given compression schemevisualization_url – url the user will need to input where visualizations will appear
- Return type:
Tuple
[Module
,CompressionStats
]- Returns:
A tuple of the compressed model, and compression statistics
Greedy Selection Parameters
- class aimet_common.defs.GreedySelectionParameters(target_comp_ratio, num_comp_ratio_candidates=10, use_monotonic_fit=False, saved_eval_scores_dict=None)[source]
Configuration parameters for the Greedy compression-ratio selection algorithm
- Variables:
target_comp_ratio – Target compression ratio. Expressed as value between 0 and 1. Compression ratio is the ratio of cost of compressed model to cost of the original model.
num_comp_ratio_candidates – Number of comp-ratio candidates to analyze per-layer More candidates allows more granular distribution of compression at the cost of increased run-time during analysis. Default value=10. Value should be greater than 1.
use_monotonic_fit – If True, eval scores in the eval dictionary are fitted to a monotonically increasing function. This is useful if you see the eval dict scores for some layers are not monotonically increasing. By default, this option is set to False.
saved_eval_scores_dict – Path to the eval_scores dictionary pickle file that was saved in a previous run. This is useful to speed-up experiments when trying different target compression-ratios for example. aimet will save eval_scores dictionary pickle file automatically in a ./data directory relative to the current path. num_comp_ratio_candidates parameter will be ignored when this option is used.
Configuration Definitions
- class aimet_common.defs.CostMetric(value)[source]
Enumeration of metrics to measure cost of a model/layer
- mac = 1
Cost modeled for compute requirements
- Type:
MAC
- memory = 2
Cost modeled for space requirements
- Type:
Memory
- class aimet_common.defs.CompressionScheme(value)[source]
Enumeration of compression schemes supported in aimet
- channel_pruning = 3
Channel Pruning
- spatial_svd = 2
Spatial SVD
- weight_svd = 1
Weight SVD
Channel Pruning Configuration
- class aimet_torch.defs.ChannelPruningParameters(data_loader, num_reconstruction_samples, allow_custom_downsample_ops, mode, params, multiplicity=1)[source]
Configuration parameters for channel pruning compression
- class AutoModeParams(greedy_select_params, modules_to_ignore=None)[source]
Configuration parameters for auto-mode compression
- Parameters:
greedy_select_params (
GreedySelectionParameters
) – Params for greedy comp-ratio selection algorithmmodules_to_ignore (
Optional
[List
[Module
]]) – List of modules to ignore (None indicates nothing to ignore)
- class ManualModeParams(list_of_module_comp_ratio_pairs)[source]
Configuration parameters for manual-mode channel pruning compression
- Parameters:
list_of_module_comp_ratio_pairs (
List
[ModuleCompRatioPair
]) – List of (module, comp-ratio) pairs
- class Mode(value)[source]
Mode enumeration
- auto = 2
AIMET computes optimal comp-ratio per layer
- Type:
Auto mode
- manual = 1
User specifies comp-ratio per layer
- Type:
Manual mode